electronics-journal.com
03
'21
Written on Modified on
Fraunhofer News
ENERGY-EFFICIENT AI CHIPS FOR ATRIAL FIBRILLATION DETECTION
AI systems can improve healthcare, increase recovery chances for patients and assist physicians in their diagnoses. The challenge is that artificial intelligence consumes an enormous amount of power. The Fraunhofer Institutes for Integrated Circuits IIS and for Industrial Mathematics ITWM have developed solutions for energy-saving AI chips that can help with early stage detection of atrial fibrillation – a special heart rhythm disorder – in the future. For their ideas, the two institutes were each awarded 1st place in the pilot innovation contest “Energy-efficient AI systems” from the German Federal Ministry of Education and Research (BMBF).
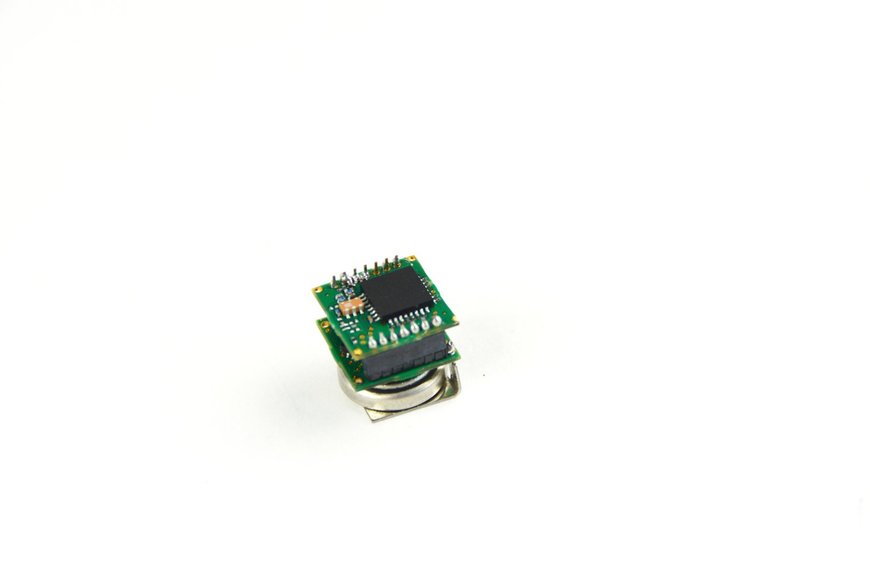
Atrial fibrillation is one of the most common types of cardiac arrhythmia. The condition can trigger a stroke if it is not detected in time. One way to record electrocardiograms (ECGs) over a long period of time, and thus increase the chance of detecting irregular heart rhythm, is to use wearables such as smartwatches that patients wear on their wrists. But for mobile diagnosis to be practical, it must be possible to analyze the recorded ECG data in an energy-efficient manner. Here's the problem: Algorithms for evaluating patient data can be very computationally intensive, which results in high energy consumption. However, the runtime of a mobile system, and thus the reliability, depends on its energy consumption. Thus the highest priority for mobile applications is the energy-efficient execution of the evaluation algorithms on the hardware.
First places for two Fraunhofer Institutes
This was why the German Federal Ministry of Education and Research (BMBF) launched the pilot innovation contest “Energy-efficient AI systems.” Artificial intelligence (AI) can only create benefits and find its way into medical, industrial and other applications if the energy consumption of today's microelectronics is reduced. The goal of the contest was for the AI chip to detect atrial fibrillation with an accuracy of at least 90 percent, to classify this in real time and to consume as little energy as possible in the process. The number of false alarms must not exceed 20 percent. To carry out the task, the Charité hospital in Berlin provided participating teams with 16,000 individual ECG recordings of two minutes long each. Of these recordings, 8000 were from patients with atrial fibrillation, the remaining 8000 were from healthy individuals. Both Fraunhofer IIS and Fraunhofer ITWM took first place, although in different categories and with different approaches. With their awards, the institutes were able to demonstrate that Fraunhofer is at the forefront of the use of AI and microelectronics in Germany.
The Fraunhofer IIS team, led by Dr. Marco Breiling, together with researchers from Friedrich-Alexander-Universität Erlangen-Nürnberg, led by Dr. Marc Reichenbach and Prof. Dietmar Fey, won in the category ASIC 130 nanometer (Application-Specific Integrated Circuit) with the project “Low-Power Low-Memory Low-Cost ECG signal analysis using ML algorithms (Lo3-ML).” Fraunhofer ITWM, in cooperation with Technische Universität Kaiserslautern, won in the category FPGA (Field Programmable Gate Array) with the project: “Holistic approach to optimizing FPGA architectures for deep neural networks via AutoML — automated machine learning (HALF).” With their victories, the two Fraunhofer Institutes have been given the chance to further develop their solutions with one million euros each.
Lo3-ML project – signal processing falls into sleep mode
To detect whether the patient is healthy or sick, researchers at Fraunhofer IIS in Lo3-ML rely on deep learning, a special machine learning method that uses neural networks with processing in multiple different layers. The digital ECG signal is used as input into the neural network, the sections of the signal are filtered, the individual signal components are weighted and summed up in several layers. The IIS researchers also refer to this as a ternary neural network, since the individual values of the time series are weighted with the ternary weight values +1, 0 and -1. “In the first layer of the neural network, a certain signal behavior is detected. In the second layer the characteristics are placed in relation to each other. A total of six layers are used. A complex image of the ECG signal that indicates an existing disease does not emerge until the last, sixth layer,” explains Marco Breiling, a scientist at Fraunhofer IIS. Breiling's research team used a trick to process these time-series signals, i.e. the digital representation of the ECG signal to enhance the energy-efficiency: They put the signal processing as a part of the AI chip to sleep as long as it is not needed. This saves 95 percent of the energy. “The chip collects the ECG signal for 12.7 seconds and then processes it in just 24 milliseconds, or 0.2 percent of the time. So the processing sleeps for over 99.8 percent of the time and uses a neglectable amount of energy. Thanks to non-volatile RRAM memories that are part of the system, signal processing can resume immediately after wake-up, after almost 12.7 seconds, without consuming any energy,” explains Breiling. The RRAMs store the ternary weights particularly efficiently.
Systolic arrays, a special chip architecture, also contribute to considerable energy savings. “For permanent operation, our chip requires so little power that a solar cell with an area of 6 x 6 mm2 operated in moonlight would suffice. Alternatively, the chip could evaluate ECGs for 330 consecutive days using the very smallest coin cell available on the market,” says the researcher. The developed circuit is not only suitable for medical use, but also for other applications where time-series signals are processed, such as condition monitoring and predictive maintenance.
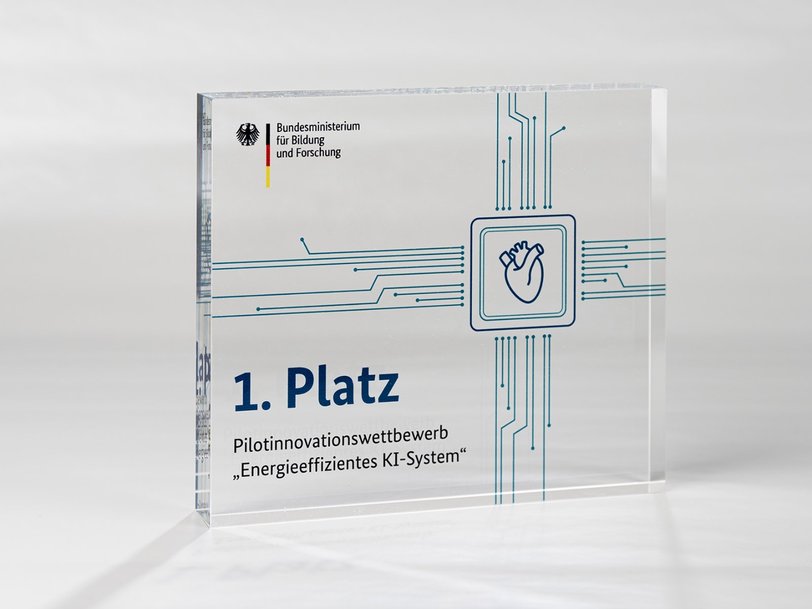
Project HALF – Holistic AutoML for FPGAs
The research team at Fraunhofer ITWM in Kaiserslautern takes both the energy consumption of the hardware and the neural network topology into account in the contest. The first step is to consider which network design offers the best prerequisites for the task. For the contest, the neural network must not only consider the accuracy of the classificaion, but must incorporate the required energy as well. It is possible to describe energy efficiency in such a way that only the minimum number of computing operations necessary are applied to detect atrial fibrillation.
AI model determines hardware energy consumption
But how exactly can the networks that meet the defined requirements and specifications be found? “There are different search strategies in this regard, and we use an evolutionary approach. We start with ten different randomly selected networks, train them and check how well they work. We then select the best networks and mutate them to create new network variants. We repeat this process until we have found the best network. This procedure is called automated machine learning,” explains Dr. Jens Krüger, who conducts research at the Competence Center — High Performance Computing at Fraunhofer ITWM and who led the project together with Prof. Dr.-Ing. Norbert Wehn from the TU Kaiserslautern. The researchers are extending this process, known as automated machine learning, to include a holistic approach that considers not only the neural network but also the hardware, since the AI model influences the energy consumption of the hardware.
Krüger and his team use programmable chips, FPGAs (Field Programmable Gate Arrays). These can map the neural networks, implement a variety of circuits and achieve the best possible execution of an optimal algorithm. The FPGA can be reprogrammed any number of times and is distinguished by various characteristics that are considered in the search for the optimal neural network. “In this respect, the project name HALF — Holistic AutoML for FPGAs — reflects the core aspect of our approach,” says the researcher. Using a software tool developed at TU Kaiserslautern, the neural network is transferred to the FPGA and is then able to automatically evaluate the ECG data. This approach has resulted in a new unifying methodology that is not only more energy-efficient than before, but also enables a reduction in development time for optimal neural network topologies and corresponding FPGA implementations. The software tools developed are suitable not only for FPGAs, but also for a wide variety of chips and environments.
www.fraunhofer.de