electronics-journal.com
03
'22
Written on Modified on
Second-Gen Habana Gaudi2 Outperforms Nvidia A100
Intel today announced that its second-generation Habana Gaudi 2 deep learning processors have outperformed Nvidia’s A100 submission for AI time-to-train on the MLPerf industry benchmark.
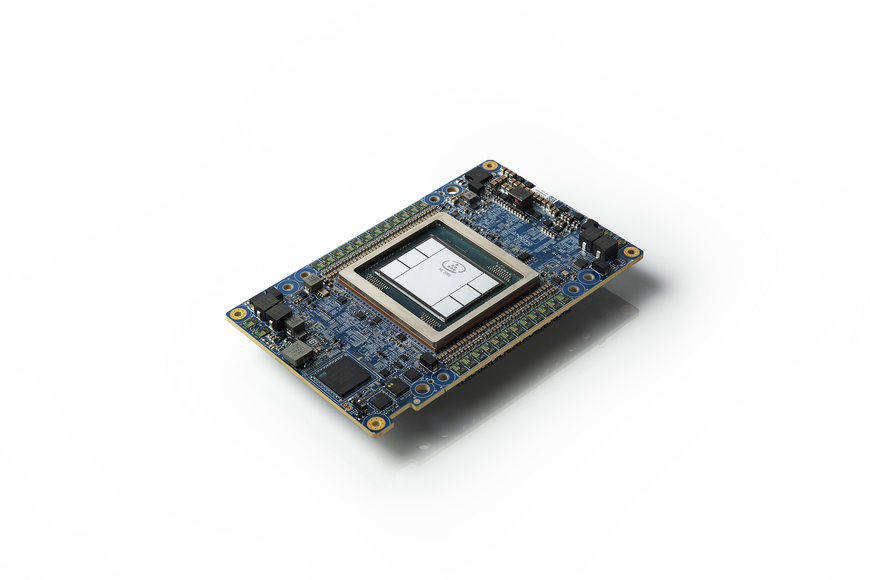
The results highlight leading training times on vision (ResNet-50) and language (BERT) models with the Gaudi2 processor, which was unveiled in May at the Intel Vision event.
“I’m excited about delivering the outstanding MLPerf results with Gaudi 2 and proud of our team’s achievement to do so just one month after launch. Delivering best-in-class performance in both vision and language models will bring value to customers and help accelerate their AI deeplearning solutions.” Sandra Rivera, Intel executive vice president and general manager of the Datacenter and AI Group.
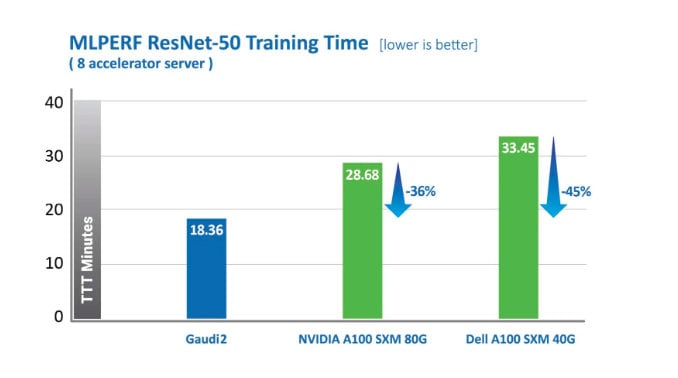
Why It Matters: The Gaudi platform from Habana Labs, Intel’s data center team focused on deep learning processor technologies, enables data scientists and machine learning engineers to accelerate training and build new or migrate existing models with just a few lines of code to enjoy greater productivity, as well as lower operational costs.
What It Shows: Gaudi2 delivers dramatic advancements in time-to-train (TTT) over first-generation Gaudi and enabled Habana’s May 2022 MLPerf submission to outperform Nvidia’s A100-80G for eight accelerators on vision and language models. For ResNet-50, Gaudi2 delivers a 36% reduction in time-to-train as compared to Nvidia’s TTT for A100-80GB and a 45% reduction compared to an A100-40GB 8-accelerator server submission by Dell for both ResNet-50 and BERT. Metrics published by MLCommons, https://mlcommons.org/en/training-normal-20/
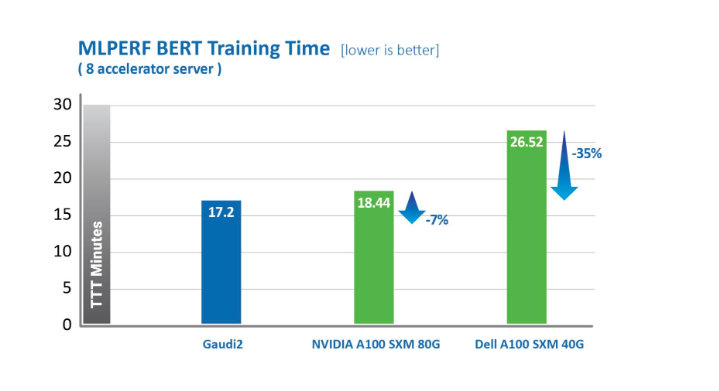
Compared to first-generation Gaudi, Gaudi2 achieves a 3x speed-up in training throughput for ResNet-50 and 4.7x for BERT. These advances can be attributed to the transition to 7-nanometer process from 16 nm, tripling the number of Tensor Processor Cores, increasing the GEMM engine compute capacity, tripling the in-package high bandwidth memory capacity, increasing bandwidth and doubling the SRAM size.
For vision models, Gaudi2 has a new feature in the form of an integrated media engine, which operates independently and can handle the entire pre-processing pipe for compressed imaging, including data augmentation required for AI training.